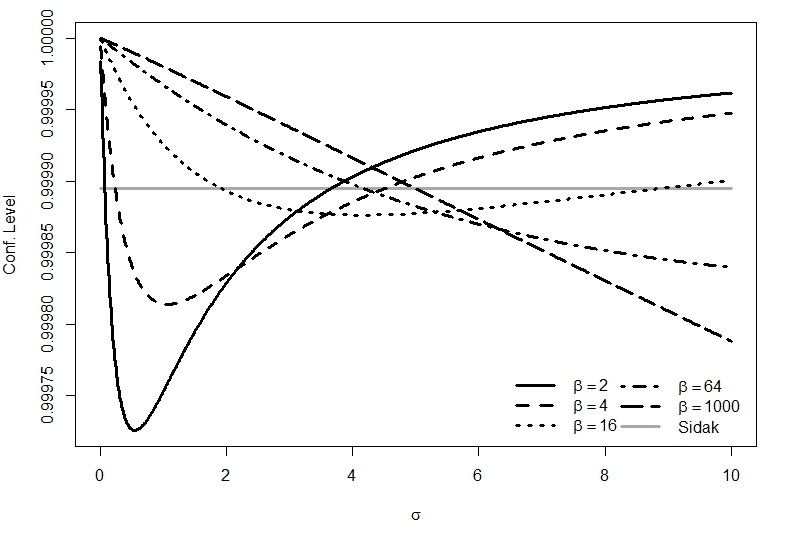
Bio Research Teaching Vitae
Dynamic Level Allocation Procedure
![]() |
Median Interval Estimation in a Nonparametric Model
This work regards the classical problem of constructing interval estimators (IE) for the median in the
nonparametric measurement error model (NMEM). The novelty of the work is the derivation of optimal
equivariant IEs on subclasses of the class of all distributions, relying only on the Invariance Principle.
The performances of the developed IEs are compared to the current methods, including the T-statisticbased
IE and the Wilcoxon signed-rank statistic-based IE, arguably the two default methods in applied
work when the target of the estimation is the center of a distribution. Applications to a real car mileage
efficiency data set and Proschan's air-conditioning data set are demonstrated. Simulation studies to
compare the performances of the different IE methods were undertaken. The results indicate that the
sign-statistic based IE and the optimal IE focused on symmetric distributions satisfy the confidence
level requirement, though they tended to have higher contents; while two of the bootstrap-based IE
procedures and one of the developed adaptive IE tended to be a tad more liberal, but with smaller
contents. However, both the t-based and Wilcoxon signed-rank statistic-based IEs should not be used
under the NMEM, as they have degraded confidence levels and/or inflated contents. |
Multiple Interval Estimation with Thresholding
![]() |
Multiple Interval Estimation for a Set of Heterogeneous Parameters
When estimating heterogeneous parameters with an MIE, one encounters difficulties in summarizing
the performance information due to the distinct characteristics of each parameter. In particular, the
expected lengths of the individual IEs require distinct measures with respect to the heterogeneous
parameters{this is like measuring apples by their circumference and bananas by their length. Therefore,
when the MIE combines these individual IEs, the global structure should involve logic to compare those
distinct measures to derive proper global performance quantities. To address this issue, I invoke the
Invariance Principle to assign groups to individual structures. Under certain conditions, the concept of
Haar measure provides the prior information for the parameter space. The resulting MIE, which is based
on the group structure, is called equivariant MIE with Haar measures (EMIE Haar). The advantage of
EMIE Haar is that the coverage probability and expected length be simplified by the Invariance Principle,
and the proper measures for the performance quantities can be derived from the Haar measure. As a
result, if group structures are available for different parameters with compatible Haar measures, the
EMIE Haar provides a coherent global structure to handle the heterogeneity. |